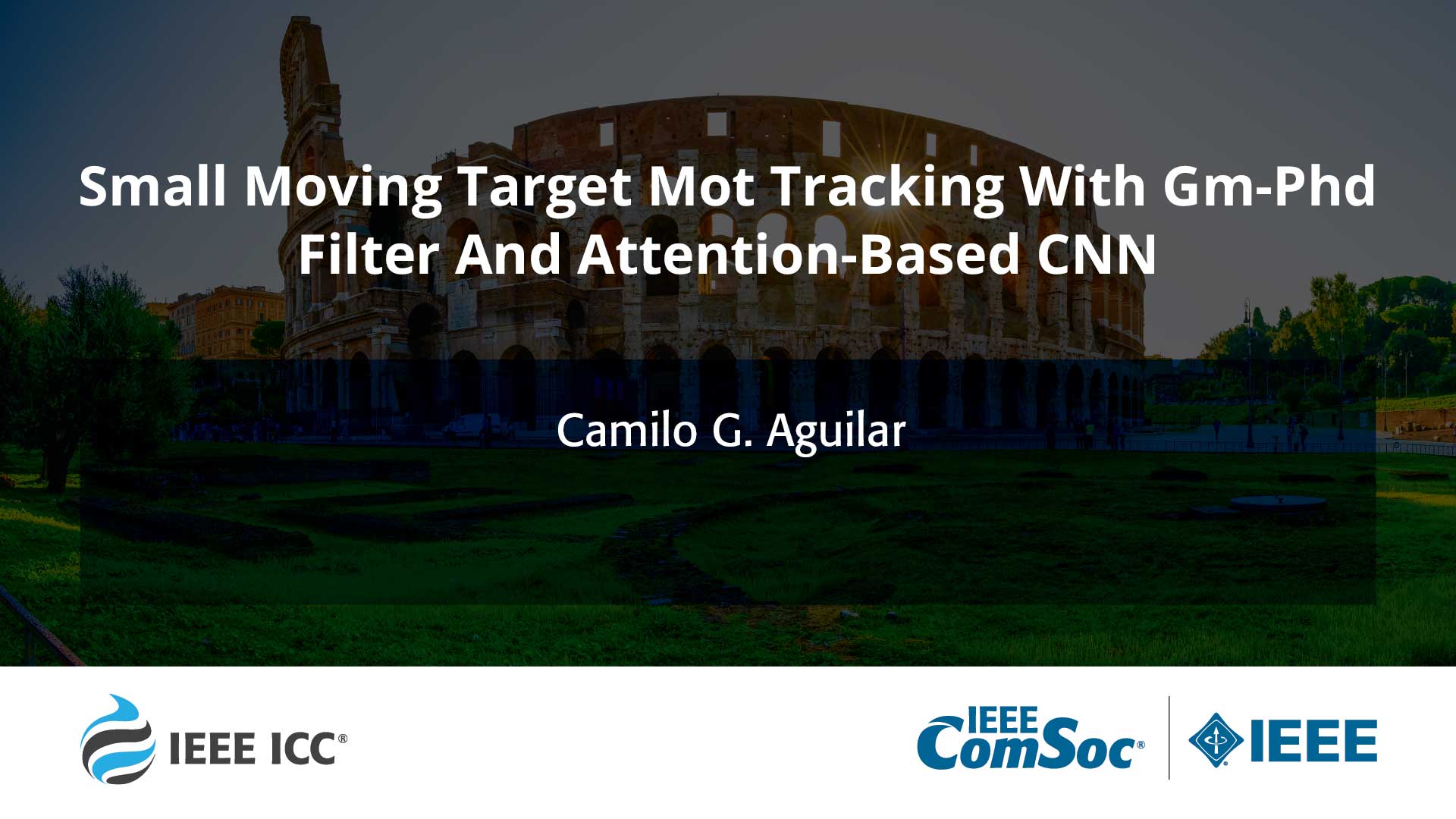
Description
The evolution to 6G promises communication-and-compute networks that are larger in scale and with significantly heterogeneous edge devices. These modern networks challenge our ability to design and optimize efficiently. While cognitive networks promise to introduce agility and intelligence, there is a need to significantly scale up such approaches in an internet-of-everything world. We present modeling strategies that effectively capture the dynamics and heterogeneity of these modern networks – however, the models come at the price of complexity. To this end, we propose a multi-pronged approach to network design and optimization. We review strategies exploiting graph signal processing for network optimization including new representations for network behavior. We show that the new representations allow for efficient graph reduction and enable low complexity optimization of network control policies. An exciting consequence is that the graph representations allow for the efficient creation of related synthetic networks, or digital cousins, that accurately capture network behavior without the need for excessive trajectory sampling of the actual network. A novel on-line/off-line Q-learning methodology is proposed enabling ensemble learning across the digital cousins. The proposed strategy offers significantly improved convergence rates and performance versus current state-of-the-art learning methods including those based on neural networks. Theoretical guarantees can be provided, and the proposed methods offer strong performance gains across a variety of networks.
Event
IEEE ICC 2023: IEEE International Conference on Communications 2023
Presenters
University of Southern California
ComSoc Member Price
$0.00
IEEE Member Price
$15.00
Non-Member Price
$25.00